Snow Crystals: A Case Study of Spontaneous Structure Formation, Kenneth Libbrecht, 2022
This is Libbrecht’s magnum opus, at least on snow; this goes deep into the science. …and I love that he has ordered the references by date, so you can see the history of the science leading up to Libbrecht’s work.
C1: Snow Crystal Science
xxx
- The most basic shape of a snow crystal is a hexagonal prism — think a hex nut but without a hole in the middle. Made up of molecules, this prism shape tends to be ‘rougher’ at the angles of the hexagon, and the roughness offers more and more favorable opportunities for H2O molecules to attach (this is in contrast to ‘flat’ or planar surfaces which do not offer such opportunities). Libbrecht refers to this as a growth instability, and it means that the prism tends to grow a projection from each angle leading to a six-pointed star and, as growth continues, a conventional snowflake shape.
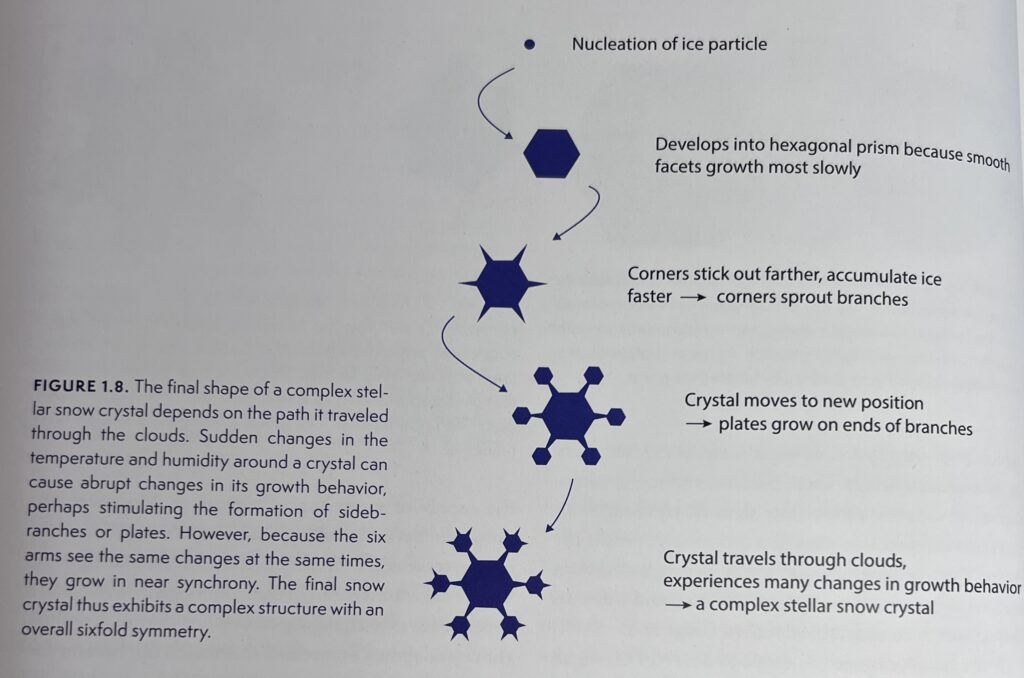
The particular form of a snowflake is finely dependent on the humidity (degree of supersaturation) and temperature. The Nakaya diagram shows the general morphologies of snowflakes that form at particular temperatures/supersaturations. Snowflake shapes are diverse, and for practical purposes, unique, because the falling flake moves through regions of the cloud that differ in humidity and temperature. Nakaya liked to remark that snowflakes are “hieroglyphs from the sky,” because their forms describe the changing conditions through which they fell as they formed.
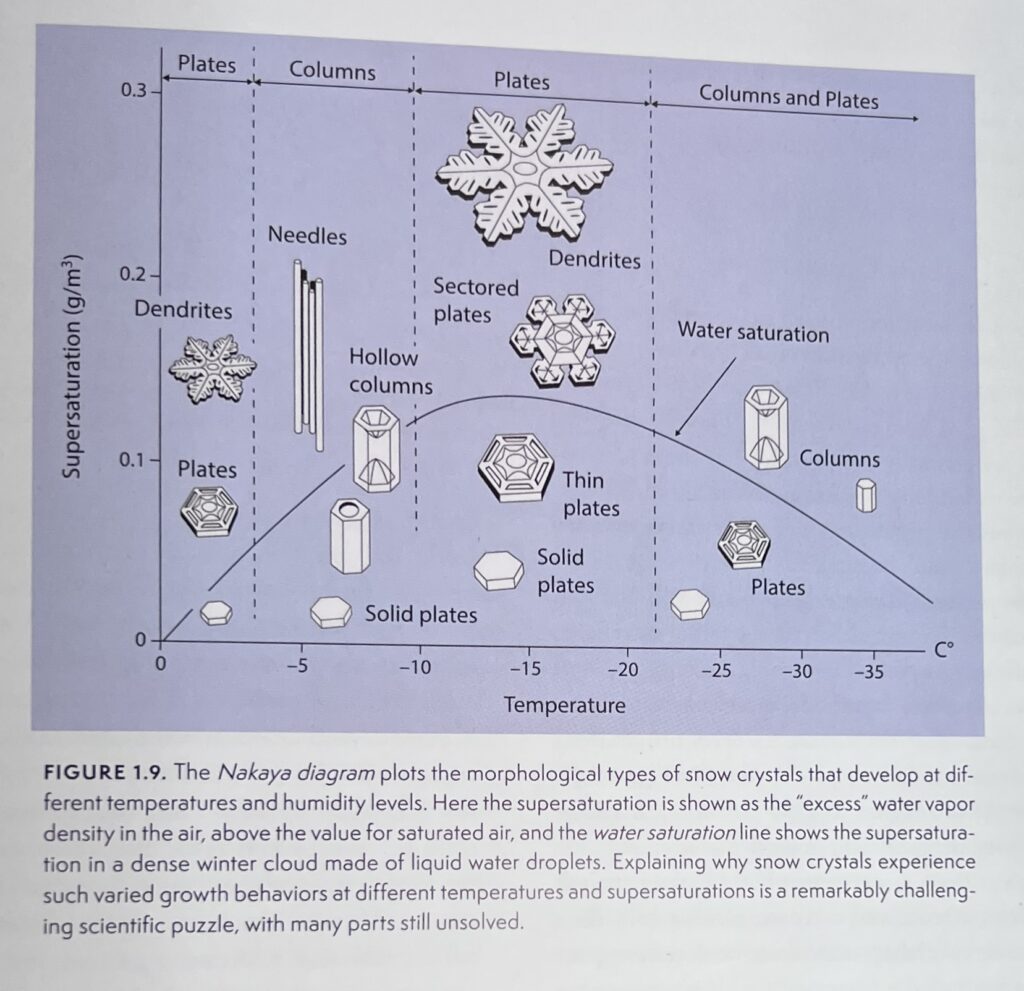
- Mullins and Sekerka realized that growth instabilities are often associated with pattern forming systems, with solidification being a specific example (See 1964Mull). In particular, a dendritic branching process, now called the Mullins-Serka instability, occurs whenever diffusion limits the solidification of materials.
- Studies of dendrite formation often involve succinonitril, because it is transparent and has a freezing point near room temperature. (See Glicksman 1976Gli.)
- Eventually work on the Mullins-Serka instability grew into an area called solvability theory, which explains many of the defining characteristics of dendritic crystal growth.
A short tour of the book
- C2: Ice Crystal Structure. Examines the properties of ice in equilibria, with special attention to “terrace step energies” in the basal and prism facets of ice crystals. Another factor called “surface premelting” is also discussed.
- C3: Diffusion-Limited Growth. The formation of elaborately branched snow crystals results from the Mullins-Sekerka instability, which arises from the slow diffusion of water vapor molecules through air. Thus particle diffusion and surface attachment kinetics are the primary physical processes guiding snow crystal growth. This line of work also shows that heat diffusion and surface energies play relatively minor roles.
- C4: Attachment Kinetics. This chapter describes the Suface Dependent Attachment Kinetics (SDAK), which along with Terrace Nucleation Theory and Terrace Step Energies can explain most of the morphological transitions seen in the Nakaya diagram.
- C5: Computational Snow Crystals. This chapter discusses work on computational models of snow crystals — this is work in progress.
- C6: Laboratory Snow Crystals. Examines various approaches to creating snow crystals in the lab.
I am going to pass on describing the other chapters… I believe that chapter 1-4 will cover most of what I’m interested in.
C2: Ice Crystal Structure
C3: Diffusion-Limited Growth
xxx
C4: Attachment Kinetics
xxx
C5: Computational Snow Crystals
xxx
C6: Laboratory Snow Crystals
xxx
C7: Simple Ice Prisms
xxx
C8: Electric Ice Needles
xxx
C9: Designer Snow Crystals
xxx
C10: Natural Snowflakes
xxx
C11: Snowflake Photography
xxx
Views: 1